Stability Optimized Exoskeleton Control
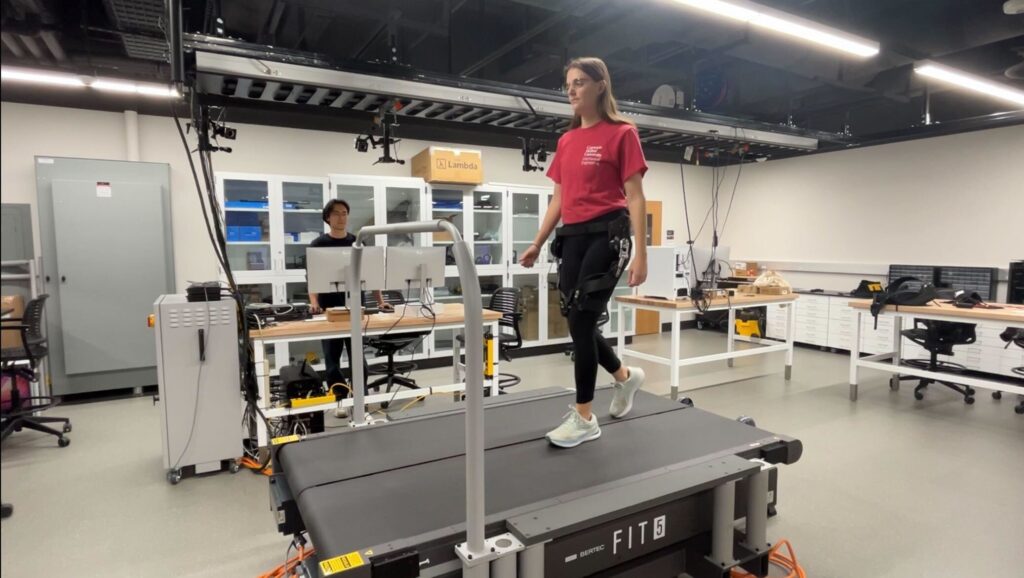
Falls during daily locomotion are a leading cause of injury among older adults, often due to delayed physiological responses to balance disturbances. While robotic exosuits offer potential for fall prevention, current strategies primarily assist movement rather than enhance stability and often rely on predefined heuristics instead of adaptive, real-time responses. Additionally, robust, generalizable perturbation detection models remain lacking. Our group aims to address these gaps by developing machine learning-driven models for perturbation detection and control architectures optimized for stability, balance recovery, and energy efficiency. Leveraging deep learning, wearable sensing, and biomechanics modeling, we aim to create adaptive stabilization strategies that enhance exoskeleton-assisted locomotion with a strong focus on real-world applicability across diverse environments and users.
Team Members
Maria Tagliaferri
Leonardo Campeggi
Pragya Devashish